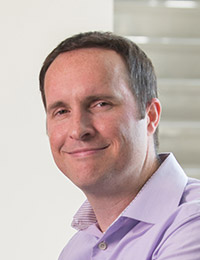
David Newton
Associate Professor of ArchitectureBrief Vitae Download CV
M.Sc. Computer Science, McGill University, 2018
M.Arch, Rice University, 2006
B.S.Arch, Arizona State University, 2001
David Newton is educated in the fields of architecture and computer science. This background informs a research and teaching agenda that merges the fields of artificial intelligence (AI) and architectural design. The resulting research agenda combines information, probability and computational theory with architectural theory to inform an information-centric approach to the study and design of the built environment. This research program is centered around the development of new critical and creative approaches to the use of data in the analysis of the built environment and in the design process that highlight the phenomenological, physiological, social, cultural, environmental and ethical dimensions of design.
His work on computation and architectural design has been published by the International Journal of Architectural Computing, the Technology | Architecture + Design Journal, AD Magazine, the Architectural Association (AA), Rice University, Actar, and Routledge. His work has also been presented nationally and internationally through the Association for Computer Aided Design in Architecture (ACADIA), Association of Collegiate Schools of Architecture (ACSA), Education and research in Computer Aided Architectural Design in Europe (eCAADe) and The Association for Computer-Aided Architectural Design Research in Asia (CAADRIA) conferences.
Research Areas
How can the allied design fields address the wicked problems posed by climate change, pollution, and scarcity of resources?
David Newton leads the Computational Architecture Research Lab (CARL) in the College of Architecture at the University of Nebraska-Lincoln. CARL aims to contribute knowledge and develop technologies that will make for a more environmentally and socially sustainable built environment, while enabling and extending human imagination and agency in the design process. The research is organized around the following areas of study:
1. Generative Methods: New conceptions of the role of information in the design process:
a. The creation of artificial intelligence-based design tools for architectural and urban design.
b. Augmented intelligence-driven architectural design tools.
2. Discriminative Methods: New conceptions of the role of information in the analysis of the built environment
a. Data analytics for architecture and urban planning:
i. Applications of machine learning for analysis and prediction of health outcomes in relation to urban morphology.
ii. Applications of machine learning for the analysis of historic urban development and its relation to sustainability.
CARL Website: https://www.computationalarchitecturelab.org
Selected Publications
Double-Blind Peer-Reviewed Journal Manuscripts
• Newton, David. “Identifying Correlations Between Depression and Urban Morphology through Generative Deep Learning.” International Journal of Architectural Computing, (May 2022).
• Newton, David. "Generative Deep Learning in Architectural Design." Technology| Architecture+ Design 3.2 (2019): 176-189.
• Richards-Rissetto, Heather, David Newton, and Aziza Al Zadjali. 2021. "A 3D Point Cloud Deep Learning Approach Using Lidar to Identify Ancient Maya Archaeological Sites." ISPRS Ann. Photogramm. Remote Sens. Spatial Inf. Sci., VIII-M-1(2021):133-139. doi: 10.5194/isprs-annals-VIII-M-1-2021-133-2021.
Double-Blind Peer-Reviewed Conference Papers
• Newton, David. 2021. "Visualizing Deep Learning Models for Urban Health Analysis." In Proceedings of the 39th Annual Education and Research in Computer Aided Architectural Design in Europe (eCAADe) Conference: Towards a New Configurable Architecture, Faculty of Technical Sciences, Novi Sad, Serbia, September 8-10, 2021, Volume 1 527-536.
• Newton, David William. 2020. "Anxious Landscapes: Correlating the Built Environment with Mental Health through Deep Learning." In Proceedings of the 40th Annual Conference of the Association for Computer Aided Design in Architecture (ACADIA): Distributed Proximities, Virtual Conference, October 24-30, 2020, 130-139. Delaware: ACADIA.
• Newton, David William. 2022. "Integrating Service-Learning Earlier in Architectural Curriculums through Engaged Design." In Proceedings of the ACSA 110th Annual Meeting: Empower, Virtual Conference, May 18-20, 2022, 85-94. Washington DC: ACSA.
• Newton, David William. 2021. "Fostering Augmented Intelligence in Architectural Education to Address Complexity." In Proceedings of the 2021 AIA/ACSA Intersections Research Conference: Communities, Virtual Conference, Sept. 29 – Oct. 1, 2021, 34-42. Washington DC: ACSA.
• Newton, David William. 2018. "Balancing Act: Techniques and Technologies for Managing Multiple-Objective Problems in a Comprehensive Studio Context." In Proceedings of the 2018 ACSA Fall Conference: Play with the Rules, University of Wisconsin-Milwaukee, Milwaukee, Wisconsin, 11-13 October 2018, 71-78. Washington DC: ACSA.
Book Chapters
• Newton, David. “Chapter 7: Deep Learning in Urban Analysis for Health.” in Artificial Intelligence in Urban Planning and Design, edited by Imdat As and Prithwish Basu, 121-137. Amsterdam: Elsevier, 2022.
• Newton, David. “Chapter 14: Dynamic and Explorative Optimization for Architectural Design.” in Routledge Companion to AI and Architecture, edited by Imdat As and Prithwish Basu, 280-300. Amsterdam: Elsevier, 2021.
Follow the College of Architecture